Unraveling the Distinctions: Generative AI vs. Other Types of Artificial Intelligence
Artificial Intelligence (AI) has been a subject of fascination and debate for decades. The field has evolved significantly since its inception, and today, it encompasses various types of AI systems, each with its unique capabilities and applications. Among these, Generative AI has emerged as a powerful and transformative force, capturing the attention of researchers, businesses, and the general public alike. This article aims to delve into the intricacies of Generative AI and explore what sets it apart from other types of AI.
Understanding Artificial Intelligence
Before diving into the specifics of Generative AI, it is crucial to have a clear understanding of what AI entails. Artificial Intelligence refers to the development of computer systems that can perform tasks that typically require human intelligence, such as learning, problem-solving, perception, and decision-making. AI systems can be broadly classified into two categories: Narrow or Weak AI and General or Strong AI.
Narrow AI systems are designed to excel in a specific domain or task, such as image recognition, speech processing, or game playing. These systems are trained using vast amounts of data and algorithms that enable them to make accurate predictions or decisions within their defined scope. Examples of Narrow AI include Siri, Alexa, and Google’s search algorithm.
General AI, on the other hand, refers to the hypothetical AI systems that can perform any intellectual task that a human can. While General AI remains a subject of ongoing research, it is still largely considered a goal rather than a reality.
Generative AI: The Basics
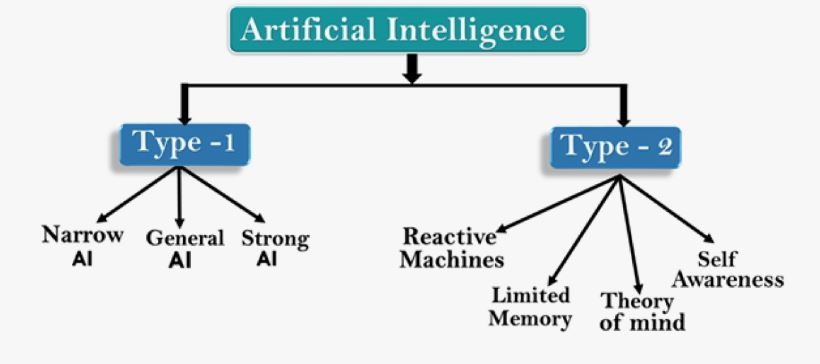
Several factors set Generative AI apart from other types of AI:
a) Data Generation: Unlike other AI systems that primarily focus on processing and analyzing existing data, Generative AI is designed to create new data. This ability to generate data has far-reaching implications in various industries, from content creation to scientific research.
b) Creative Capabilities: Generative AI systems can mimic and even surpass human creativity in generating new and original content. This capability has led to exciting advancements in fields such as art, music, and literature.
c) Model Learning: Generative AI models learn by understanding the underlying patterns, structures, and relationships within the data. This learning process enables them to generate new content that is not only similar to the input data but also displays a level of originality and creativity.
d) Applications: Generative AI has a wide range of applications, including natural language processing, image and video generation, text-to-speech conversion, and even drug discovery. Its versatility makes it a valuable tool in various industries, from entertainment to healthcare.
e) Challenges and Limitations: While Generative AI has shown remarkable progress, it still faces several challenges and limitations. These include the need for large amounts of high-quality data for training, potential issues with data privacy and security, and the difficulty in ensuring the generated content’s accuracy and reliability.
Comparing Generative AI with Other Types of AI
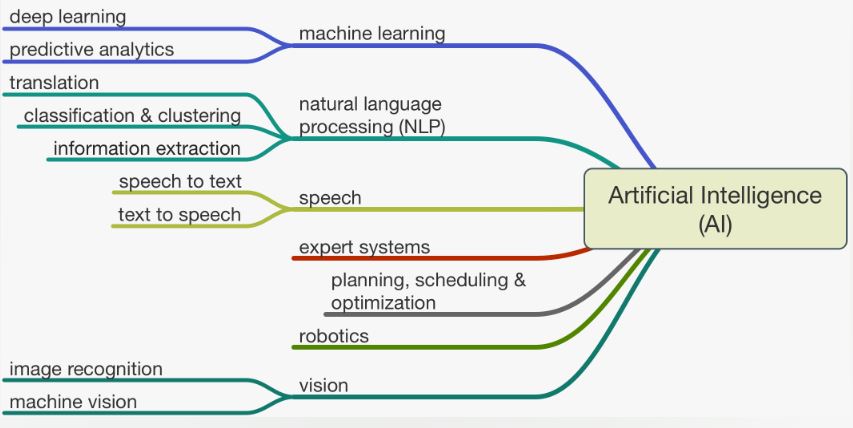
To better understand what sets Generative AI apart, it is helpful to compare it with other types of AI:
a) Narrow AI: While Generative AI and Narrow AI both utilize machine learning techniques, the primary difference lies in their objectives. Narrow AI systems are designed to solve specific problems or tasks, whereas Generative AI focuses on creating new data or content.
b) Deep Learning: Deep Learning is a subset of machine learning that uses neural networks to learn from data. Generative AI often employs deep learning techniques to generate new content. However, deep learning can be applied to various AI tasks, not just content generation.
c) Reinforcement Learning: Reinforcement Learning is another type of machine learning that involves training AI systems to make decisions by learning from the consequences of their actions. While Generative AI can use reinforcement learning, the primary focus remains on data generation rather than decision-making.
The Future of Generative AI
Generative AI’s rapid advancements and diverse applications have led to widespread excitement and anticipation for its future. As the technology continues to evolve, we can expect significant developments in various industries, including:
a) Content Creation: Generative AI will likely revolutionize content creation across various mediums, enabling the rapid generation of high-quality text, images, videos, and music.
b) Personalized Experiences: Generative AI’s ability to create customized content will enable businesses to offer tailored experiences to their customers, enhancing engagement and satisfaction.
c) Scientific Research: Generative AI’s potential to generate new data and hypotheses will accelerate scientific research, leading to breakthroughs in various fields.
d) Healthcare: Generative AI can play a crucial role in drug discovery, medical image analysis, and personalized medicine, significantly improving patient outcomes.
Conclusion
Generative AI stands out as a unique and transformative force within the realm of artificial intelligence. Its ability to generate new data and content, coupled with its creative capabilities, sets it apart from other types of AI. As Generative AI continues to evolve and mature, it will undoubtedly reshape industries and revolutionize the way we interact with technology, ultimately enhancing our lives in countless ways.
FAQs
Generative AI focuses on creating new data or content, while other AI systems primarily process and analyze existing data.